ISyE Associate Professor Yao Xie is aiming to create higher confidence in the data models that drive societal outcomes
By David Mitchell
In countless fields from law enforcement to medicine and many in between, professionals look to make educated predictions about outcomes based on available data.
Consider police who may want to find two crimes committed by the same person. Can they find the needle in the proverbial haystack of countless police reports? How about a doctor trying to make decisions that will prevent patients from encountering sepsis? Can they parse enough data – blood pressure, blood sugar, temperatures, heart rate, and more – at various times to come up with a prediction with high enough confidence levels to help inform a doctor’s potentially life-and-death decisions?
Current approaches typically rely on statistical models, but those are often simplified to avoid plummeting confidence levels. A new study by researchers in Georgia Tech’s H. Milton Stewart School of Industrial Systems and Engineering (ISyE), and led by Yao Xie, associate professor in the school, offers a new approach using deep learning that could change the way decisions are made in similar situations.
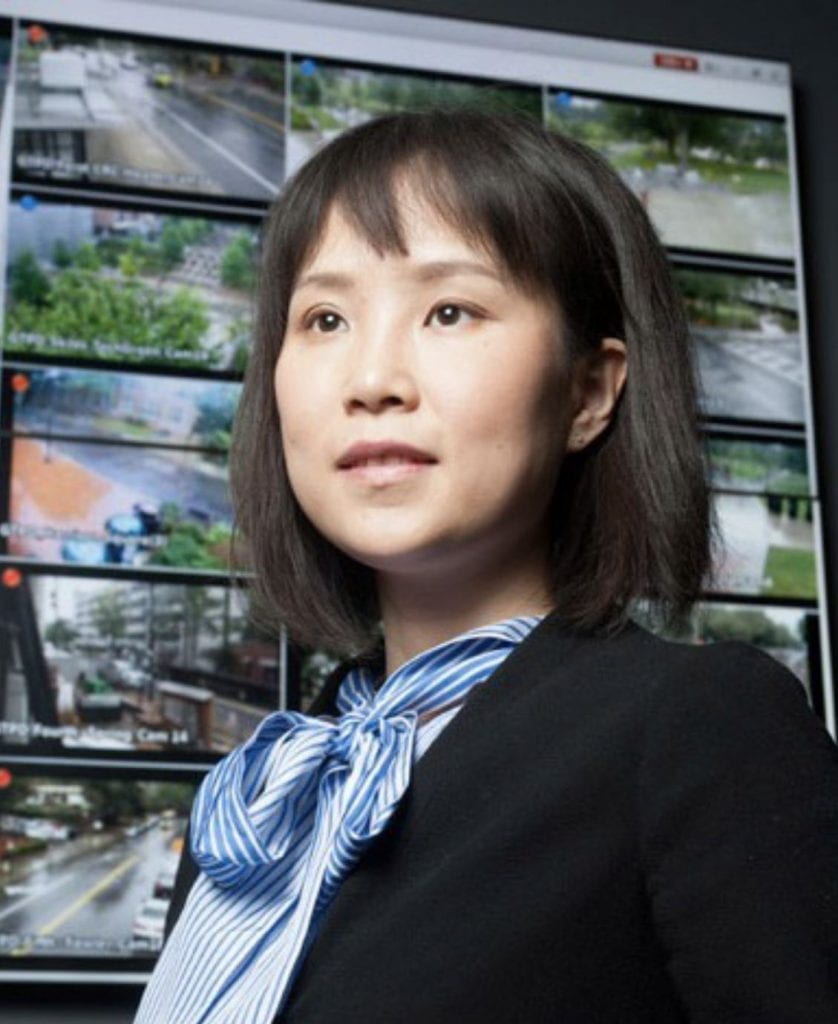
The paper, which is published at the International Conference on Learning Representations (ICLR 2022), advocates for the use of machine learning to go beyond standard point process data that classifies events at certain points in time. Using a neural network’s advantage in efficiency and ability to parse larger amounts of data, researchers are able to look at non-stationary data – like blood pressure, heart rate, or temperature in a patient – to model more potential outcomes at higher levels of confidence.
“Sepsis is one of the most dangerous conditions that will lead to death quickly once it happens,” said Xie, the Harold R. and Mary Anne Nash Early Career Professor in ISyE and associate director of the Machine Learning Center. “We would like to collect information about the patients’ health conditions together with tests and results and outcomes so that we have a sequence of observations over time. This contains so much information about the patient and, with that, we can come up with a prediction score to help inform a doctor whether the patient is safe or at risk of sepsis.”
This work is derived from a large $1.1 million grant from the National Science Foundation that is helping her lab explore new ways to improve the use of deep learning models to predict future outcomes.
“Machine learning can be beneficial because it can parse such large amounts of data,” Xie said. “But they often lack guarantees. We are trying to bridge the gap such that we can extend the use of deep learning by providing performance guarantees.”
The key to advancement, she said, is the robust optimization of these models as well as advancements in mathematical understanding of deep learning theories – essentially, how algorithms are trained.
Potential use cases for this approach are widespread. Beyond health care and law enforcement, it has application in weather, geological occurrences, clinical trials, and supply chain.
The paper is titled Neural Spectral Marked Point Processes, and is written by Shixiang Zhu, Haoyun Wang, Zheng Dong, Xiuyuan Cheng, and Xie.